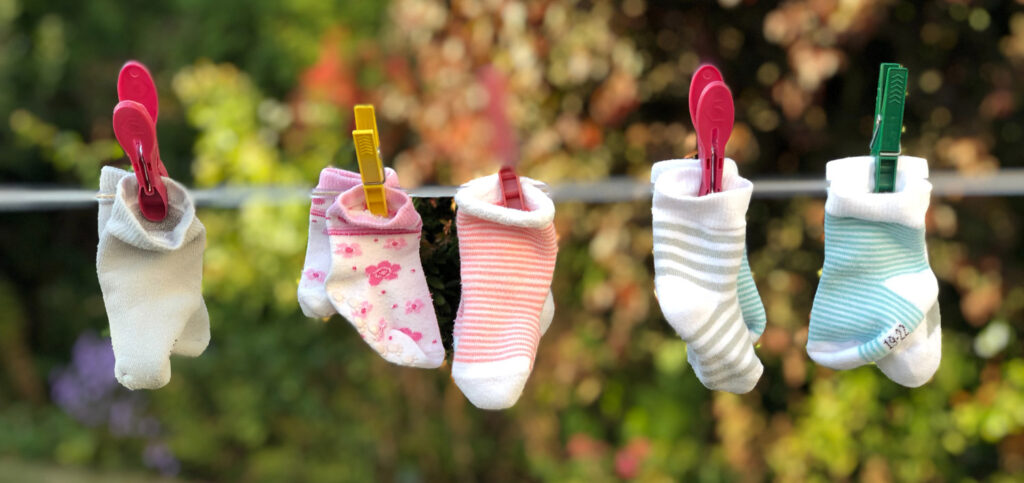
A blog article by Ashlie Reker Ph.D.
Pair Matching is a method of splitting study subjects into experimental groups in a way that limits the possibility of independent variables skewing the study results.
To run a scientific study using Pair Matching, study subjects are placed into experimental groups based on specific blocking variables. This allows for equal distribution of the blocking variables across all experimental groups, which is crucial to the validity of the data collected and subsequent experimental conclusions.
Why Pair Match?
Pair Matching is intended to control for effects of confounding variables, ie, a factor that affects both the dependent and independent variables in such a way that false associations can be created between the two. These associations can result in incorrect experimental conclusions. Each confounding variable must be controlled for across all experimental groups in order to draw accurate conclusions from experimental data.
Example Study without Pair Matching
Let’s look at an example experiment that does not use Pair Matching to create the experiment groups. We want to assess the effect of Compound-Z on weight loss versus placebo control using a sample of 8 people.
The 8 people are split randomly into two groups, as seen below.
At the end of our study, both groups lost about the same amount of weight- indicating that Compound-Z is no more effective at promoting weight loss than the placebo. However, since we employed a completely randomized design, we ended up with significantly more males and a lower average age in our placebo control group.
There is evidence to suggest that men lose weight more rapidly than women ( Christensen, P., et al., 2018; Wing, RR & Jeffery, RW; Williams, R.L, Wood, C.E., Callister, R, 2014) and that younger people lose weight more rapidly than older people (Marie-Pierre St-Onge, Ph.D, Dympna Gallagher, Ed.D., 2010) Therefore our results may have been skewed simply because there were more males and a younger population in our placebo control group!
These data leads us to conclude that Compound-Z is not effective at promoting weight loss; however it is also possible our results were skewed by the confounding variables of sex and age. An incorrect conclusion could lead us to abandoning further research on Compound-Z and depriving patients of a critical medical treatment.
Let’s Try This Again with Pair Matching!
Let’s run our experiment again, this time using Pair Matching to ensure both experimental groups are sex and age matched. In this example, we are blocking the confounding impact of sex and age. This results in both groups having roughly the same number of males and females and approximately the same average age. We start by matching subjects by sex and age into pairs and then randomly allocating the individuals to an experimental group, as illustrated below.
Now that our study groups are Pair Matched, we know that the confounding variables of sex and age are evenly distributed across both our study groups. We can be confident in the accuracy of our data and the conclusions drawn from our study results!
For a real-world example, oncology studies often use Pair Matching to create their experimental groups. In vivo models of cancer have pre-determined acceptance criteria set for tumor volumes that have to be met for an animal to be enrolled on study. Due to the variability in tumor growth rate, the criteria is usually a range (for example 100-200mm3). Within the set range, animals should be distributed so that the same tumor width x length combinations are present in all experimental groups.
Can I do Pair Matching in Climb?
Climb 2.0 can accommodate Pair Matching methodology! Having Pair Matching functionality in Climb allows scientists to create experimental cohorts all within in a single platform. All animals available for study enrollment can easily be randomized into cohorts, housed, and enrolled on study to begin data collection. The critical values for the blocking variable(s) are saved as part of the animal and cohort metadata, making reporting and reproducibility possible with a few clicks.
If you want to utilize Pair Matching in your studies or have questions about Climb’s capabilities, contact us and one of our experts will be in touch!